Image
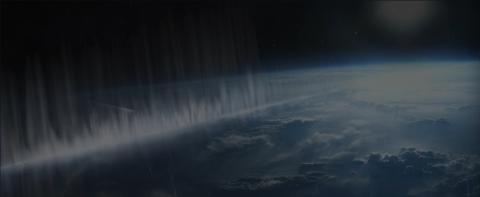
Geophysicists heavily rely on software-based mathematical models to map and characterize features beneath the Earth’s surface. These models face challenges throughout the lifecycle of the model, from data gathering, to input assumptions, to data denoising and interpolation, to data output, to model validation. It is widely acknowledged that virtually all geophysical models are burdened with non-uniqueness. The numerous complexities and limited ability to access the object being modeled, in this case the Earth, open the door for assumptions that, when coupled with an infinite number of possible outputs, can hamstring the model’s accuracy and usefulness. An important discussion is warranted to assure that models are properly vetted to prevent misinterpretation, which leads to misrepresentation, and to ascertain their effectiveness, particularly given the reliance on models as a tool in exploration or as evidence for a given hypothesis or theory. Geodynamic modeling is especially susceptible to the inherent shortcomings of modeling, with a higher bar required to achieve usefulness. The appeal to geophysics models in defending plate tectonics is widespread among young earth creationist geologists. With a broader picture of the geophysical modeling methods presented, young earth creationists are cautioned to be mindful of the pitfalls of modeling, which too often is oversold or misrepresented. In the case of catastrophic plate tectonics, forward models appear too unconstrained — the constraints applied are subjective or unrealistic — to yield substantive conclusions about the validity of the proposed catastrophic mechanism.
Models are used to simplify and approximate an otherwise complex real-world system. It may be a representation of a specific structure or object, or a simulation of the action and behavior of a system. Models are especially necessary in geophysics, as the Earth’s interior cannot be effectively observed. The ultimate goal of geophysical modeling is to attain the best possible approximation of geological structures or processes from geophysical data. These data are typically the measured signals of gravity or magnetic fields, or electromagnetic or seismic waves, which have been filtered through a set of structures within the earth. The model then inverts these signals to find characteristics of this earth filter, and thus discerns the set of causal structures.
Geophysicists widely acknowledge the inherent problem of model non-uniqueness, where the measurable data are insufficient to triangulate or constrain a particular solution. However, non-uniqueness may not be the only challenge. During the lifecycle of the model additional influences occur, such as noise amplification and poor convergence that can degrade or destroy the model’s image of a geological structure. In some instances the limitations are rigorously mitigated such that a reasonable case can be argued for the model’s usefulness in predicting sub-surface structures. This is particularly true when borehole data are used as feedback to constrain the solutions of a seismic inversion model that maps rock strata. However, models without the constraints of external feedback and rigorous validation mechanisms are characteristically of much lower confidence and may be of little use, being dominated by uncertainty.
In this paper we primarily focus on two models: Seismic wave analysis (inverse modeling), and plate tectonic models (forward modeling). Seismic tomography is the most widely used by geologists and others in related disciplines, which is used to create a three-dimensional model of subsurface structures based on the velocity and paths of seismic waves. It is used in academia and by corporations, especially in the oil and gas industry.
Plate tectonic modeling is primarily limited to academia and small research projects.
Imaging models used to map subsurface areas include seismic tomography, seismic reflection, and seismic refraction imaging. These imaging techniques heavily rely on inverse modeling in its iterative process to analyze the travel times and paths of acoustic seismic P and S waves. The software-based inversion model depends on complex mathematics to decipher wave behavior and map possible representations of the object as images (inverse modeling seeks to best approximate the input given known output data such as wave measurements and earthquake foci).
Seismic tomography is primarily used in academia to map deep internal structures in the mantle and core. The P & S waves are sourced by natural events, particularly earthquakes, and recorded at stations around the globe for later analysis involving inverse modeling. It provides the widest scope of the different imaging techniques.
Seismic reflection (seismic imaging) is used to map shallow depths and is triggered using controlled sources such as explosives or seismic vibrators. The oil and gas industry heavily relies on seismic reflection when searching for natural resources. Whenever feasible, these companies will leverage model constraints that may include magnetic and gravitational data, and/or borehole data as a feedback mechanism into the inverse model to improve model accuracy (Engheim 2018).
Seismic refraction provides details of the shallowest layers by including refraction analysis of the seismic waves. Similar to seismic reflection, controlled sources are used to generate seismic waves. Seismic refraction is used for near-surface examination that includes groundwater exploration and determining suitability of construction sites.
The steps for a seismologist to construct an image of the subsurface of an area is a long and arduous task. It is a process that consists of data acquisition, processing and interpretation. Errors in any of these phases can significantly impact the final output. The latter two phases of processing and interpretation have been equated to scientific art based on experience. The seismologist must compile data on travel times related to the shot and receiver positions, remove travel time based on surface conditions, attempt to assess and remove noise, remove response times of receivers, deal with spatial resolution, and much more. As two geophysicists lamented in their blog “We seismologists, however, make countless choices, approximations and assumptions, which are limited by poor data coverage, and ultimately never fit our data perfectly. These things are often overlooked, or taken for granted and poorly communicated. Inevitably, this undermines the rigour and usefulness of subsequent interpretations in terms of heat or material properties.” (Koroni 2019)
Seismic imaging techniques are often compared to inverse modeling used to produce image maps in computerized tomography (CT) scans via X-rays, and magnetic resonance imaging (MRI). However, seismic wave output is much more prone to inaccuracy than CT or MRI scans due to a litany of factors. These include 1) fewer wave paths, 2) curved wave paths, 3) unknown source location, 4) limited or no constraints (eg, no well data), 5) high variation in properties such as permeability and density, 6) limited model validation, etc. (Julian, 2006).
Moreover, inverse models are notoriously non-unique, especially given the ambiguity of the subsurface being modeled. As noted in the textbook Introductory Geophysical Inverse Theory: “There are almost always many possible answers to an inverse problem which cannot be distinguished by the available observations” (Scales, 2001). Given an inverse model’s inherent susceptibility to non-uniqueness, finding reasonable model constraints to mitigate the problem is essential. The goal is to restrain interpretations as much as possible using independent data sets. Geophysicists nevertheless generally acknowledge that inverse models are under-constrained (Pinet 2019). Poorly constrained models with even a single data misfit will invariably yield an infinite number of models, which is essentially no model at all. Multiple constraints and cooperative inversions are often used to isolate the most plausible quantitative best-fit of the input data (Reid 2014). For example, gravity and magnetic data can be used as a weak constraint on seismic models, but they typically measure different sets of properties and at a lower resolution.
In seismic wave analysis, constraint data that is paramount to improving inverse model accuracy are borehole data. Without them, the model will be ill-conditioned and prone to subjective interpretation: “It is well known that seismic tomography is an ill-conditioned inverse problem due to limited illumination of the medium. Borehole data as constraints is essential for the important task of producing seismic images that tie the well” (Gosselet, 2009).
Academia is replete with peer-reviewed articles describing plate subduction which appeal to seismic tomography imaging that has limited constraints or feedback parameters, such as what may be provided by borehole data. Without adequate controls, model uncertainty is high, so caution is warranted before accepting or promoting claims in articles of academia using weakly constrained seismic imaging data. For example, when 20 independent geophysicists analyzed seismic data from subsurface faults in the Hoop area in the southwestern Barents Sea, there was considerable variation in interpretation, locating fault tips was difficult and smaller faults were rarely recognized, and machine-learning fault interpretation provided little improvement in clarity (Faleide, 2021). In a recent study of five seismic models of the Chinese mainland, the researchers found that “large discrepancies exist between the models, both in absolute values and perturbation patterns” (Zhang 2022).
When borehole data are available to academia, they are typically only from a handful of distant wells. One such example is imaging of the subsurface of the northern Hikurangi subduction zone in New Zealand (Gray 2019). Three boreholes were referenced, U1518, U1519, and U1520. The deepest of the three is U1520 at roughly 1000 meters (Wallace 2019). Given the limited number and depth of the wells reasonably calls into question the overall strength of these constraints, and even with possible disparity mitigation, it can only assist as a feedback constraint in shallow subsurface regions.
Where you will find multiple borehole data constraints is in the geophysical engineering world of the oil and gas industry. These corporations are in the money-making business, where the incentive and accountability for seismic imaging accuracy is much greater. They engage in proprietary and more advanced full waveform inversion (FWI) seismic imaging (SLB.com 2014, ExxonMobil.com 2018). They do not do research on plate boundaries (pers. comm. Neal Secorsky, Senior Geophysicist & Supervisor at Schlumberger, June 2022). But even so their more advantageous technology would likely not provide a significant boost in confidence of results sought to bolster evidence for subducting plates (Pinet 2019). Ruslan Miftakhov, chief technical officer of GeoplatAI, acknowledges that “the success rate of oil and gas drilling operations remains low, even after implementing expensive technical analysis and procedures” (Miftakhov, 2021). Despite rigorous efforts in the oil and gas industry where seismic accuracy is much more critical, it is clear that borehole feedback is not a panacea and enormous challenges remain.
Consider the failures of seismic wave analysis when deep borehole data became available via the Kola Superdeep Borehole project and the German Continental Deep Drilling Programme. Russia’s Kola borehole is the deepest in the world in terms of pure vertical depth at 12.3 km, which is only a fraction, roughly 1/4th, of the average depth of continental crust. Germany’s KTB borehole reached 9 km. Both highly publicized projects yielded dramatically unexpected results inconsistent with prior seismic modeling. In the Kola borehole, seismic analysis had previously predicted a transition from granite to basalt at roughly 7 km below the surface due to seismic wave velocity anomalies. Instead, the borehole revealed crushed granite saturated with water (microscopic plankton fossils were also found, which further confounded scientists). Regarding the KTB borehole in Germany, the Journal of Geophysical Research reported that “The geologic starting model proved to be inaccurate… Likewise unexpected, and of considerable consequence with respect to geologic models based on surface mapping, is the intense brittle deformation and the enormous amount of thrust faulting” (Emmermann 1997). The JGR report also noted “A highly unexpected result, confirmed by a number of independent observations, is the lack of any P-T gradients down to at least 8000 m and the uniformity of radiometric ages”. As summarized in the Wiki article German Continental Deep Drilling Programme, “…it had been expected that the large tectonic pressures and high temperatures would create metamorphic rock. Unexpectedly the rock layers were not solid at the depths reached. Instead large amounts of fluid and gas poured into the drill hole.”
Subjective wave analysis is often misleading when varying velocities are associated with a specific property such as temperature. As noted by the USGS, “many factors affect the wave speed, including composition, crystal orientation, mineralogy and phase (especially the presence of melt). Red anomalies may not really be hot, nor blue ones cold” (Julian, 2006). While many scientific articles properly reference seismic images in a context of wave velocity, many do not. A popular seismic image of alleged plate subduction that is commonly referenced online and in creation literature promoting catastrophic plate tectonics (CPT) is shown in Figure 1. The image, which originates from a seismic study a quarter century ago (Zhao 1997), is limited to questionable earthquake foci constraints (Zhao 1997, footnote 9). Despite dated technology and the lack of additional constraints, assumptions are made that the blue colors represent a “colder ocean lithosphere,” or “colder subducted slabs” (Clarey 2018, 2020). What is the result is an image that may be giving an illusion of subduction. As noted in the Canadian Journal of Earth Sciences, “some end-users… put more confidence in attractive images, in a way similar to the customer’s reaction to an advertising campaign” (Pinet 2019).
Another study that has been referenced in support of CPT (Clarey 2016, 2020) provides seismic tomography of what is an alleged subducted plate beneath the continental United States (Schmandt 2014). This is also speculative given that the article also failed to provide constraining data beyond earthquake loci. While this paper did not use language assuming “cold” lithosphere and properly attributed the blue areas to “high-velocity anomalies”, it nevertheless succeeds in giving the illusion of “cold” by shading the inferred slabs in their tomography images as blue (Figure 2).
Another example of subjective interpretation of subducting plates is shown in Figure 3. This seismic tomography image from geophysicists at The University of Texas at Dallas used the more-advanced FWI coupled with a geophysical measurement referred to as seismic anisotropy (Zhu 2020). This academia-based study is also missing important borehole constraints. But even if we assume that the images are fairly accurate, it is apparent that claims of subducting plates are subjective, and any flood model proponent could find evidence for and against the hypotheses formed using this image. For example, the authors note that the Caribbean slab dips from 30 degrees to 90 degrees, straight down, and attribute the steep dip of the Cocos slab to greater age (23 Myr) and therefore greater density. The slabs are in pieces, and several are detached from the surface. It would be a tenuous (and circular) claim to stake this tomography as evidence for plate tectonics.
Another case of oversimplification and questionable speculation from seismic models stems from the image in Figure 4, which is commonly used among creationists when promoting catastrophic plate tectonics (CPT). In this case the low-velocity anomalies are attributed to warmer rocks, and through additional assumptions the temperature differences between warm and cold rocks are on the order of 3,000 to 4,000 Kelvin (Baumgardner 2003). This is postulated as a problem for the uniformitarian framework and support for CPT (Baumgardner 2003, Hebert 2017, Clarey 2018, 2019), and even as a prediction of CPT (Ham 2021).
The two oversimplified blobs in Figure 4 refer to two widely known subsurface anomalies geophysicists have observed from seismic data since the 1970s that lie on roughly opposite sides of the Earth, one beneath Africa and the other below the Pacific Ocean (Duncombe 2019). More recent seismic depictions of these large low-velocity anomalies are shown in Figure 5. The two regions of lower-than-average shear wave velocities are referred to as low shear velocity provinces (LLSVP).
A lot of liberty in interpretation must be taken to extrapolate these LLSVPs into evidence for CPT. There are at least three problems with this:
(1) A wide range of interpretations are available, so deciding on one and using it as evidence for a flood model is overly premature. Mineral physicist Dan Shim from Arizona State University notes that “not knowing the density leaves many doors open” (Duncombe 2019). Phil Heron and Ed Garnero in their review of the LLSVPs in The Geological Society eloquently describe the many possible interpretations that have been made of the blobs, such as contradictory postulations on the LLSVPs’ material density to misinterpretation of what might simply amount to “thin, thermal plumes with smeared resolution at depth.” They conclude: “The diversity of these interpretations throughout the geophysics community highlights the difficulties in constraining mantle dynamics” (Heron, P. & Garnero, E. 2019).
(2) Secular geophysicists generally do not appeal to these anomalies as evidence of plate tectonics, which CPT is based on, so neither should creationist geophysicists! As noted in The Geological Society review, “despite advances in analytical techniques and increased data volumes, the causes of this structural complexity and links with mantle dynamics and plate tectonics remain open questions” (Heron, P. & Garnero, E. 2019).
(3) Geophysicists have known of these anomalies since the 1970s, before CPT was proposed, so claims that CPT a priori predicted these alleged “cold” remnant oceanic plates is false (Ham 2021).
Forward modeling imitates a system by applying inputs to a simulation or complex algorithm and observing the output. Such modeling is often conveyed by the simple expression d = F(m), where ‘F’ are the governing equations, ‘m’ is the model, and ‘d’ are the predicting data. Forward modeling is used throughout the geosciences, and often plays an important role as a tool to assist in hazard mitigation of earthquakes, volcanoes, movement of contaminants in groundwater, etc. This paper focuses on geodynamic modeling, a type of forward modeling that attempts to mimic large-scale processes in the Earth, such as plate movement, plume flow, fault zone behavior, mantle convection, etc. Such models may constitute physical or numerical modeling, wherein physical principles and computational methods are used (Van Zelst 2022).
Given the extreme complexity of the Earth, which has a virtually unlimited range of geophysical subsystems with complex spatial and temporal interactions, there is an inherent litany of uncertainties with geodynamic models that attempt to simulate geophysical motion in the Earth. Hence the adage “all models are wrong, some are useful” is especially germane to geodynamic models. The ultimate question is: Can the model reasonably produce useful information? That is, can the model reduce uncertainty in a hypothesis that the model seeks to quantify? While models are intended to represent simplifications of reality, to be useful and predictive they must reasonably account for system properties (e.g., mineralogy), motion physics, intra-dependencies (e.g., impact of temperature and pressure on rheological structures), and inter-dependencies (e.g., impact of gravity and magnetism). Synchronous and asynchronous events and the order in which they occur also must be reasonably accounted for.
The modeling approach this paper addresses is forward modeling of plate tectonics, which itself employs numerical modeling, requiring solutions to equations. The most popular method in geodynamic numerical modeling is the finite-element method (FEM). There is also a growing number of geophysicists exploring the less-complex finite-difference method (FDM) and the finite-volume method (FVM). Examples of tectonic modeling using FEM include TERRA (Baumgardner 1994, 2018) and UnderWorld2 (Moresi 2021). Models using FDM include the LaMEM code (Almeida 2022).
Within engineering, FEM is used to assist in product design. A computer simulation that applies the mathematical equations behind FEM into a visual representation is referred to as a finite element analysis (FEA), which requires proper interpretation to constrain or identify weaknesses in a design. WiseGeek appropriately describes FEM as follows:
“The finite element method is a tool for computing approximate solutions to complex mathematical problems. It is generally used when mathematical equations are too complicated to be solved in the normal way, and some degree of error is tolerable. Engineers commonly use the finite element method because they are concerned with designing products for practical applications and do not need perfect solutions. The finite element method can be adapted to varying requirements for accuracy and can reduce the need for physical prototypes in the design process.”
“The Earth is an incredibly complex system on which a large number of forces act generating a flurry of geological and geophysical phenomena occurring over a wide range of spatial and temporal scales” (Zaccagnino 2022).
While it is well understood that geodynamic modeling faces difficulties and challenges unlike most other models, the enormity of these problems cannot be overstated. They are covered below:
The influence of factors such as gravity and wind can displace mass within the Earth and on the surface (Dumberry 2022). Geodynamic modeling necessitates consideration of associated physics, which should include the conservation of mass, energy, and momentum.
Rheology, which describes the deformation of material under stress, must be properly leveraged in geodynamics models. This is a gargantuan task that subjects any model to extremely complex interdependent interactions of variables that describe rocks and their behavior when stress is applied. To make matters worse, the Earth's rheology is not well known (Crawford 2016).
In addition to internal dependencies and interactions, complexity is added by external inputs that may be synchronous (e.g., the moon’s gravity causing tides), or asynchronous (e.g., wind, water motion, erosion, chemical reactions).
Equation of state is a thermodynamic equation based on physical conditions as dictated by state variables such as volume, pressure, temperature and energy. Many state variables relevant to mantle behavior are largely beyond our reach (Bokulich 2017).
Governing equations for the physical processes are needed, including equations for the conservation of mass, energy, and momentum, rheology, fluid dynamics, and domain geometry (Van Zelst 2021).
Modeling plate tectonics requires assumptions on driving mechanisms for plates and lithospheric slab subduction, such as slab pull as the driving force of plate motion. It is questionable if any geodynamic theory adequately explains plate motion (Olson 2010, Zaccagnino 2022).
More variables mean the model will become more computationally intensive and require greater computer power.
There is scant direct observational data of the Earth’s subsurface. This affects the ability to find useful model constraints and a means to verify the model.
There are numerous other complex processes that require consideration, including generation of the magnetic field in the outer core, two-phase or multi-phase flow, disequilibrium melting, complex magma dynamics in the crust, reactive melt transport, dehydration reactions and water transport, mineral grain size, anisotropic fabric, phase transformation kinetics, and inertial processes and seismic cycles (Van Zelst 2021).
In addition to the afore-mentioned challenges, which help paint a picture of the enormity of the task to develop a model to imitate these complex interactions and dependencies, it is also important to keep in mind the vast number of assumptions that must be made for input parameters to feed the model, as well as assumptions on internal constant variables (e.g., equations of state). Without sufficient knowledge of the assumptions (as inputs to the model and as constants within the model), the usefulness of the model in supporting or contradicting a hypothesis is highly questionable. Associated geodynamic model pitfalls include:
Models should aim to be of the simplest form that produces the best fit. As variables are added to a model, complexity increases, especially when the variables influence each other. Variable dependency is measured in statistical regression modeling as R2, which is the proportion of the dependent variable’s variance as explained collectively by independent variables. As more variables are added, R2 increases or stays the same, but never decreases. A higher R2 generally indicates a better fit to data. However, adding variables also increases the likelihood that the model will mold itself around the data and produce unreliable output. For example, focused scope such as groundwater modeling provides an example of modeling that with proper inputs can provide some information that can be useful in planning and mitigation. However, too many details can result in unwieldy and inaccurate results (Provost, A 2010). Models that employ machine learning increase the likelihood of overfitting (Liu 2022). Machine learning attempts to mitigate this with training data, which is used to tune the algorithm to best-fit output (called the Train-Test Split procedure).
Improperly vetted input variables and a model’s internal constants can greatly limit the accuracy of output. In the paper Finding a suitable finite element for 3D geodynamic modeling, the authors lament that FEM models “usually contain large abrupt viscosity variations, and mixture of compressible and incompressible material behavior... Almost entire 3D finite element codes employed in geodynamic modeling community use some form of spatial discretization that violates the mathematical criteria of stability, the so-called Ladyzhenskaya-Babushka-Brezzi (LBB) condition. The explanation behind this fact is that instabilities do not necessarily show up in practice, but unstable discretization is relatively computationally inexpensive... In the Rayleigh-Taylor benchmark with large (>1000) abrupt viscosity contrasts, discretized with non-fitted mesh, the meaningless velocity solutions can be obtained even with stable elements” (Popov 2010).
Determinism yields the same result given the same inputs, and conversely non-determinism is a property whereby the same inputs can produce different outputs. The finite element method is inherently deterministic because it employs spatial discretization. However, geodynamic processes are inherently non-deterministic. Geodynamic models that use determinism therefore exclude an important aspect of geo-processes, thereby increasing the uncertainty of the output. But if they include non-deterministic state changes the range of outputs exponentially increases, which can also increase uncertainty of the model output absent reasonable validation tests.
Given the limited observational data, model testing and validation is extremely challenging, and is in fact rarely included in the exploration cycle (Pinet 2019). The lack of sufficient validation is arguably the biggest Achilles heel of geodynamic modeling. This will be addressed in greater detail in the next section.
A paper on geodynamic modeling does a good job of describing the full life-cycle of a geodynamic model, as shown in Figure 6. This section of the paper focuses on the Validation portion of the model lifecycle. Model verification and validation is an important part of any software application and is critical for performing its intended requirements accurately and with stability. A typical validation process is: 1) bench testing, 2) unit testing, 3) peer-review of code changes, 4) white/black-box testing, 5) regression testing. These processes will be covered below and include a discussion of their effectiveness in the context of geodynamic inverse and forward modeling.
The first validation step in the software development process is the developer manually confirming that the application, new feature, or bug fix does what it is intended to do. In the engineering world bench testing is expected and almost universally practiced.
Unit testing is a software development process in which smaller parts of an application are independently validated. The software developer is typically responsible for writing unit tests to validate the new feature they coded. This step aims to identify potentially fatal bugs early and helps assure full code coverage via testing. As the developer writes the test to validate their own software, such tests are typically not a strong line of defense for validating the code, and often-times it becomes busy work rather than improving the quality of a product. Unit tests are part of a methodology called Test Driven Development (TDD), which is intended to improve software quality while also better familiarizing the developer with adjacent parts of the code base.
In the context of general engineering programs, it is this author’s opinion as a lead developer with experience over a diverse number of successful projects that unit testing is more often than not a counterproductive use of time, and its overall effectiveness in improving software quality at the cost of productivity and time-to-market is debatable. Studies to this effect confirm the author’s suspicion of unit test’s mixed results (e.g., Madeyski 2010, Munir 2014). Unit testing is seldom used in smaller teams that may be commissioned to be efficient and innovative with a critical deadline to meet. Ideally, unit testing should only be targeted where it makes reasonable sense to include it. This assumes that the software will still be tested via much more effective black-box and regression testing, which is virtually always the case within engineering companies.
In the context of complex numerical modeling, such as what is used with geophysical inverse or forward modeling, a good case can be made that unit tests are worthwhile, especially in sections of the code that involve complex and not previously vetted mathematical algorithms. This would not be necessary for algorithms that have stood the test of time, such as those already available in a code library. Coding platforms typically have math and date libraries that are well proven and are thread-safe. Examples include the GNU Scientific Library (GSL) and the Intel MKL library. Nevertheless, inverse models or geodynamic models that piecemeal functions from mathematical libraries that are then used to calculate some new mathematical output would warrant unit-testing of that section of code.
Most software processes include a code review step before code is allowed to move forward to a test verification team. Once a developer finishes an application, feature, or bug fix, and — if required by their team — completes any associated unit tests, the code is put up for review. There are software packages that aid in this process, such as Code Collaborator. Code repositories such as BitBucket and GitHub provide lightweight code review tools.
The effectiveness of code reviews depends on many factors, including the number of lines of code to review, the size of the review team, the desire and ability to review code, and social factors. Code review requires a special skill that only a handful of developers possess, not for lack of inability but for lack of desire. Studies generally conclude that code reviews when exercised properly are only slightly worthwhile (Bacchelli 2013, Czerwonka 2015). This author agrees that code reviews are typically “less about defects than expected and instead provide additional benefits such as knowledge transfer, increased team awareness, and creation of alternative solutions to problems” (Bacchelli 2013). This author also agrees that when not implemented properly, code reviews provide an overall negative impact due to product delays that outweigh benefits of the code review (Czerwonka 2015).
White-box testing, also referred to as clear-box or transparent-box testing, is a technique that has knowledge of internal code structures and is given a means to validate it, typically via an API designed to provide the access. This is often useful to test complex portions of code that may otherwise require extensive black-box or regression testing to catch intermittent corner cases.
Geophysical inverse modeling and geodynamic forward modeling would especially be fertile ground for white-box testing because of their inherent complexity with numerical modeling.
The most important and effective validation method is black-box testing, which is a form of testing that does not know the internal structure or code paths of the application being tested but understands the requirements and use cases the application is supposed to support. Black-box testing primarily focuses on validating output based on input. This may include software-based tests that exercise a range of inputs and use cases, then check for results based on those inputs. It is generally preferred that such tests are deterministic, so that a test run that produces unexpected results can be reproduced. Also common is manual “visible” testing of the application to confirm use cases. Examples of black-box testing include writing data to an SSD drive then reading it back and confirming the data, driving a vehicle to confirm GPS tracking is working, running structural software and manually confirming that the output meets the required standards and conforms to the laws of physics.
Negative testing is also an important component of black-box testing, where invalid or unexpected inputs are supplied and the application is checked to see if it gracefully handles the data. This may include reordering of input parameters or asynchronous inputs. Examples of negative testing are error injection in a NAND model and GPS out-of-range behavior in vehicle tracking software.
Black-box testing is especially necessary to find corner-case problems that bench and unit testing are not geared to find. There may be intermittent problems where algorithms work most of the time, but not under some scenario based on a specific sequence of events or inputs. An example from Micron was a corner case where a mathematical algorithm worked the vast majority of time, but a very intermittent miscalculation was caused by improper typedef casting and was only exposed under heavy test load.
Regression testing typically involves a full suite of manual and automated unit, white-box, and black-box testing. This phase of testing is important beyond just validating the product, but to also maintain application stability as bug fixes and new features are added.
Typically before software is released, potential consumers of the software may be asked to run the application with the knowledge that it is pre-release and in the final stage of validation.
Once software is released, there will be some level of ongoing maintenance required, based on bugs reported by users or new feature requests.
Unfortunately, black-box validation, which is the most critical to determine model accuracy and reliability, is extremely limited with seismic imaging software and geodynamic forward modeling. It is a significant weakness that is virtually unavoidable given the complexity and limited access to that of what is being imaged, the Earth’s subsurface. The closest seismic imaging can get to black-box testing is when attempts can be made to match seismic imaging to the Benioff zone of earthquake foci. This type of validation is extremely limited given that the deepest borehole is only a fraction of the Earth’s crust (Stierwalt 2020). The deepest boreholes near alleged subducting plates are just 3 km into the crust (Notman 2022). The cost for drilling boreholes is also enormous, averaging $200,000 to $800,000 per day of drilling (Richter 2022). Even with borehole data, challenging disparities remain between extracted borehole data and seismic images (Emmermann 1997, Boughton 2013, Fundytus 2022).
With geodynamic modeling, there may be cases where a historical event such as a local flood can be compared with model predictions of water flow, but such data are rare and difficult to quantify. And even when available, the models still show low fidelity. For example, in a 2001 study, the authors showed that many hydrological models fail because of “unanticipated changes in the forcing functions of the systems they represent. More broadly, ‘validated’ models may fail for the following reasons: First, systems may have emergent properties not evident on smaller scales; second, small errors that do not impact the fit of the model with the observed data may nonetheless accumulate over time and space to compromise the fit of the model in the long run; and, third, models that predict long-term behavior may not anticipate changes in boundary conditions or forcing functions that can radically alter the system's behavior” (Bokulich 2017).
Geodynamic models are further limited in validation and vetting given that they invariably originate from very small teams (often just one or two-man teams), see insubstantial use outside their group, and provide either completely inadequate or no validation data (Ghosh 2019, Bercovici 2014, Baumgardner 2018, Almeida 2022). Even when some of these models are publicly available as open source, the developers are often within academia, which usually means they have less programming experience and practice weaker source control. The geodynamic open-source Python-based software Underworld2 claims to have a “broad network of collaborators,” but a quick glance at their GitHub shows just how limited its exposure is, with currently only one occasionally active contributor (Underworld2 GitHub 2022). Another application used in a peer-reviewed science article that attempted to characterize plate movements is the model LaMEM (Almeida 2022). The GitHub repo for this model also reveals low developer activity, and similar to Underworld2 also uses Python as their programming language. Python is a beginner’s-choice language that has poor performance, which makes it a questionable choice for complex modeling that if done properly should be hungry for CPU power (Python is a run-time compiled and interpreted language that is inherently slower than C, C++, Java, C#, etc).
Some geodynamic models use Fortan, which is a far superior choice and well-suited for extensive numerical calculations and offers good performance. While Fortran clearly gives a model better credibility than those developed with Python, it does not rescue the model from an absence of black-box validation. Consider open-source database software PostgreSQL, which should pale in complexity to geodynamic modeling software. It is exposed to multiple users across the world, which has resulted in multiple releases that include major bug fixes. Micron’s NAND model was developed by a dozen engineers and was vetted by rigorous regression testing and large engineering teams, requiring multiple releases and ongoing maintenance. At MiTek, their materials and structural modeling software, called MiTek Structure, engages half-a-dozen engineers on their maintenance team alone to deal with new bugs that arise from unexpected real-world corner cases (Interview with Ryan Williams, Team Lead of Software Assurance, MiTek, 2022).
To further illustrate the problem of small or one-man teams limiting proper software vetting, consider Dr John Baumgardner’s paper Numerical Modeling of the Large-scale Erosion, Sediment Transport, and Deposition Processes of the Genesis Flood (Baumgardner 2018). The author only defends validation of the model’s internal mathematics: “The equations commonly used to model such flows are anchored in experimental measurements and decades of validation in many diverse applications.” While accurate mathematics is an important component of any model, this does not validate the overall model (called MABBUL), which was designed to simulate the transport and deposition of sediments worldwide. This is akin to appealing to a specific bench or unit test of an internal code section as the only form of validation. It is generally expected that internal libraries are already well-vetted. Micron NAND model’s internal Linux libraries are trustworthy because of decades of use in many diverse applications across the globe, but this does not mean the NAND model itself has been validated. Validation of internal libraries alone would never be accepted in the corporate engineering world as a demonstration that the model produces useful output. Black-box testing and customer exposure are the ultimate barometers to determining the overall veracity and fidelity of an application or model.
Baumgardner later defends validation of the model by appealing to its output, saying that it closely matched what has been observed with global sediments (Baumgardner 2021). This type of validation is essentially bench testing only, which is susceptible to single-user bias and by even the loosest of engineering standards is wholly insufficient to qualify the model. Models such as this risk falling into a circular trap in which empirical data are either used to build the model, or, when the data fail, to calibrate the model against different scenarios (Bokulich 2017). Aside from inadequate testing, given all the afore-mentioned challenges with geodynamic modeling with an almost unlimited number of assumptions and possible outputs, the likelihood that the MABBUL model provides supportive evidence of slab motion causing sufficient energy to deposit all the world’s sediments is tenuous.
Achieving useful modeling for complex simulators is a slow process. For example, high-fidelity vehicle and flight simulators take years of man-hours and development, with ongoing fine tuning from real-world feedback and unanticipated use or corner cases. The relatively high fidelity of flight simulators was not possible without validation and feedback based on actual flight data (Pavel 2013). Less-complex modeling such as Micron’s NAND model also requires months of development, testing, and upgrades/fixes based on ongoing validation and unanticipated use/corner cases. Proper device programming, error handling, and voltage threshold distribution tuning cannot be validated without known reference data from actual NAND hardware. Even with access to real-world data, numerous challenges remain in adequate modeling of NAND technology (Bennett 2016).
For geodynamic models, feedback is either non-existent or extremely limited. Unlike industry models such as flight simulators, construction modeling, and NAND modeling, geodynamic models that attempt to mimic major processes such as plate tectonics do not have an established template or historical events to compare them against. Such models are virtually void of any constraints or test mechanisms to provide reasonable confidence in their accuracy. Consider that it took decades of complex mathematical modeling and computer programming, validated against real-world data, before flight simulators were promoted to limited use in training (Pavel 2013, FederalRegister.gov 2016, Saastamoinena 2021). As reported in Procedia Computer Science, “simulator training is an important part of flight training, but it is equally important to teach flying in practice. As an example of this, when the US Air Force gradually reduced the flight hours of KC-135 pilots on the right aircraft and increased simulator flight hours in basic training, reduction saved costs, but as a result, pilots could not land properly on a real plane” (Saastamoinena 2021). The Air France 447 disaster in 2009 was partially blamed on training reliance on a flight simulator’s improper handling of a specific in-air scenario of how swept-wing aircraft react when they exceed the critical angle of attack (Mark 2019). After decades of validation and real-world feedback data, modern flight simulators have demonstrated the latter half of George Box’s adage on models that while “wrong,” they certainly meet the criteria of usefulness.
But can the same be said for geodynamics models? In assessing their usefulness, further consideration must be given to the environment in which they are developed and validated. As previously mentioned, geodynamic models are developed almost exclusively either within the confines of academia or in small research projects that do not fall under the umbrella and supervision of a significant body of accountability. Within academia, while there is some level of accountability, e.g., performing on grant money or earning a good grade on a project, it pales in comparison to accountability within an engineering company, where deadlines must be met at the risk of missing important time-to-market windows that could jeopardize company profits and the very jobs of the developers on the project. Validation standards in the corporate world are much more rigorous, where the vast majority of companies involved in software development have their own dedicated independent test teams. Furthermore, the level of expertise in software development is far greater within software companies than academia. While many computer science professors have real-world experience, their students do not. In this author’s experience, even the best college grads will not see critical path work on a project until they have at least one year of experience, and it typically takes five to 10 years of experience before a developer is trusted with lead or architecture responsibilities. It is simply not realistic to expect a similar standard of excellence and fidelity in a computer program from the confines of academia when compared with companies engaged in software development, where there is far more at stake, and where the vast majority of invention and innovation occur.
But is all hope lost for geodynamic models being “useful”? While it may be virtually impossible to develop a useful geodynamic model to mimic plate movements with a reasonable level of confidence, it is well within reach to prove the negative; that is, a model that provides a data point that demonstrates the impossibility for a certain outcome. To illuminate this point, consider Mendel’s accountant, a model designed to emulate population genetics within a neo-Darwinian framework of natural selection plus random mutation (Baumgardner, Sanford et. al 2018). On the one hand, the model faces challenges similar to geodynamic modeling given its inherent complexity with virtually unlimited input variability, in addition to its limited validation and vetting within a small research group. The authors make a highly questionable assertion on the model’s usefulness as a “capable research tool that can be applied to problems in human genetics, plant and animal breeding, and management of endangered species.” This is an overstatement of the model’s capability, especially given the minimal verification metrics acknowledged by the authors that are merely based on other mathematical models, not real-world data. Moreover, important and far-reaching inputs are not considered, such as the impact and role of epigenetics, which poses a significant challenge in its own right and is an ongoing and lively debate among creationists (Guliuzza 2019, Lightner 2019). Mendel’s accountant does however have a clear advantage over geodynamics models given its potential to improve forward predictive value by tapping into population genetics data as a template for validation, especially with organisms with short reproductive cycles.
On the other hand, Mendel’s accountant provides compelling evidence against a neo-Darwinian framework that posits overall genetic improvement. As noted by the authors “If one uses parameters corresponding even in a crude sense to observed biological reality, then Mendel always shows genetic deterioration, not genetic improvement” (Sanford et al, 2008). Only when non-natural (miraculous) inputs are used, such as identical fitness effects of mutations and truncation selection, does the model prevent genetic deterioration. A similar narrative occurred with a numerical model called ‘Ev’ that was developed by NIH scientist Dr Tom Schneider when he was a grad student at the University of Colorado. Dr Schneider, who has used information theory in his genetics research on binding sites, claimed that his Ev simulation demonstrated how Shannon information can increase at the genetic level. In personal correspondence, he unwittingly admitted to extreme truncation selection in his Ev program due to his inexperience with software (Williams 2005).
Physicist Richard Feynman said it best: “In the natural physical sciences, it is difficult, some might say impossible, to prove a proposition to be true; but, on the other hand, it is quite possible to prove that which is false.” Mendel’s accountant sufficiently demonstrated that while its predictive ability for organism adaptation and population growth is highly questionable, it succeeds in proving that genetic improvement using very favorable assumptions for evolution is not possible without the help of the non-natural (aka miracles). Likewise, Dr Schneider’s Ev program proved increased genetic information via random processes was not possible without invoking the same truncation selection miracle.
We contend that while the numerical models of Mendel’s accountant and Schneider’s Ev program are “wrong,” they are useful in demonstrating that which is false, something that mathematicians have known all along — random mutation and blind selection invariably leads to deterioration of genetic information. By extension, we contend that geodynamic models are wrong, but have the potential to be useful if geared properly to proving that which is false. When considering Baumgardner’s geodynamic TERRA modeling, if we apply the same standard as when assessing Mendel’s accountant and Schneider’s Ev program, TERRA’s only reasonable usefulness is that it might refute that which the model sought to demonstrate — CPT. Indeed, TERRA requires several miracles for it to work. For example, “the physical laws were somehow altered by God to cause [plate tectonics and the flood] to unfold” (Baumgardner 1990). Also needed are colder lithospheric slabs: "An initial temperature perturbation is required to initiate motions within the spherical shell domain that represents the Earth’s mantle. For this, a temperature perturbation of -400K to a depth of a few hundred kilometers is introduced around most of the perimeter of the supercontinent” (Baumgardner, 2002). Also needed is a mushier mantle: "The mantle’s viscosity at [the time of the flood] was lower than at present to permit rapid sinking of the lithosphere into the mantle” (Baumgardner 1990).
In response to a featured article in the US News and World Report in which TERRA modeling was featured, the National Center of Science Education (NCSE) countered with the article “Miracles In, Creationism Out” (Matsumura 1997). Can we reasonably argue that they were not justified in their objections? Just as we cannot allow evolutionists to use miracles to defeat Mendel’s accountant and save Neo-Darwinism, we should not allow creationists to use TERRA miracles to save CPT.
Information is the reduction of uncertainty. Before a model is used, there is an initial level of uncertainty in its ability to reasonably achieve what it is designed to mimic. Consider MRI or CAT scan modeling software, designed to visualize parts inside the body to look for bone fractures, blood clots, tumors, etc. Without a validation process, uncertainty would remain high. However, with the ability to surgically validate imaging, which also serves as valuable feedback for model enhancement, uncertainty in the fidelity of the scans is significantly reduced. Uncertainty in the ability for flight simulators to emulate real-world scenarios is reduced due to in-air pilot experience and flight recording data serving as confirmation feedback that continually improves flight simulator fidelity.
Geodynamic modeling, given extremely complex inter and intra-system interactions with virtually no access to feedback for proper validation, inherently retains a high level of uncertainty between model input and output. This is particularly true for global-scale tectonic plate models such as TERRA, MABBUL, and Underworld2. These complex models are virtually void of validation methods and simply cannot reasonably meet a standard of usefulness as a forward model.
The amount of time spent implying that TERRA and more recently MABBUL modeling provide evidence for CPT should alarm creationists. Research papers and numerous lectures, often in their entirety, have been dedicated to promoting models that are highly likely to be wrong with no explanatory power. Moreover, the TERRA model has been compared to corporate models despite a stark and clear dissimilarity. Any model that appeals to non-natural processes would not be accepted in the oil and gas industry, and such models would never see the light of day in the engineering world.
While ongoing innovation in tomographic inverse modeling continues to improve subsurface knowledge, these incremental improvements represent only a fractional gain on the scale of model effectiveness and accuracy. When real-world seismic data and observation are compared to model-based predictions, the success rate remains low where it matters most, with the oil and gas industry that pours millions of dollars and man hours into this research. In academia, the problem is exacerbated, as models have limited access to important constraints such as borehole data, no access to superior proprietary subsurface technologies, and limited or no real-world experience in seismology. In the context of flood geology, even with the most advanced tomography, a reasonable case cannot be made that supports one flood model over another (e.g. subduction vs collapse); the evidence lies in the subjective eyes of the beholder. We therefore urge creationists to view such tomographic-based studies with caution and recognize the weaknesses outlined in this paper so that seismic inverse modeling is not oversold as evidence supporting one flood paradigm over another. We also discourage unbridled use of “hot and cold” in creationist literature when referencing tomography data, as any number of factors can contribute to wave velocity.
Geodynamic modeling that attempts to mimic plate movements faces an even greater litany of challenges ranging from limited knowledge of rheology, thermodynamic variables beyond our reach, virtually unlimited inter and intra-dependencies, and insufficient ability to validate model outputs against historical, observable, or even current real-world data. Such models also exist primarily in academia and small research teams, where standards of excellence inherently cannot reach the level achieved in the oil and gas industry that spends billions of dollars on fully dedicated research teams that have more experienced geophysicists at their disposal. Application of geodynamic models with realistic inputs may prove useful in stressing edge conditions to determine if a paradigm can withstand them, that is, to ascertain if the paradigm will not work. But the uncertainty that geodynamics can simulate plate movements is too high — such a model’s outputs would be deemed unreliable and would not meet even minimal standards in the engineering world. Geodynamic models are undeniably hamstrung at no fault of their own when compared with most other engineering models, but this should not become a free pass to ignore the elephant in the room. Creationists should therefore dismiss news reports and studies from academia that champion geodynamic modeling.
Because geodynamics modeling used to support catastrophic plate tectonics includes supernatural inputs, modeling output cannot possibly provide useful information. Such a position represents a classic trap of circular logic. Creationists should therefore steadfastly reject geophysical forward modeling used as evidence for catastrophic plate tectonics.
Almeida, J., Riel, N., Rosas, F. 2022. Self-replicating subduction zone initiation by polarity reversal. Communications Earth & Environment. DOI: 10.1038/s43247-022-00380-2
Bacchelli, A., Bird C. 2013. Expectations, Outcomes, and Challenges of Modern Code Review In: Proceedings of the 2013 International Conference on Software Engineering. ICSE ’13, 712–721. IEEE Press, Piscataway. http://dl.acm.org/citation.cfm?id=2486788.2486882.
Barker, D., S. Henrys, F. Tontini, P. Barnes, D. Bassett, E. Todd, L. Wallace, 2018. Geophysical Constraints on the Relationship Between Seamount Subduction, Slow Slip, and Tremor at the North Hikurangi Subduction Zone, New Zealand. Geophysical Research Letters, Volume 45, Issue 23, Pages 12,804-12,813. https://doi.org/10.1029/2018GL080259
Baumgardner, J. 1990, Creation Research Society. CRSQ 1990 Volume 27, Number 3, pp. 98-100. https://www.creationresearch.org/crsq-1990-volume-27-number-3_the-imperative-of-non-stationary-natural-law
Baumgardner, J.R. 1994. Computer modeling of the large-scale tectonics associated with the Genesis Flood. In Proceedings of the Third International Conference on Creationism, ed. R.E. Walsh, pp. 49–62. Pittsburgh, PA: Creation Science Fellowship
Baumgardner, J. 2002, Catastrophic plate tectonics: the geophysical context of the Genesis Flood. Journal of Creation. Volume 16, Issue 1:58-63.
Baumgardner, J.R. 2003. Catastrophic Plate Tectonics: The Physics Behind the Genesis Flood. Proceedings of the Fifth International Conference on Creationism. R. L. Ivey Jr. (Ed.), pp. 113–126, 2003.
Baumgardner, J., Sanford, J., Brewer, W., Gibson, P., and ReMine, W. 2008. Mendel's Accountant: A New Population Genetics Simulation Tool for Studying Mutation and Natural Selection. The Proceedings of the International Conference on Creationism: Vol. 6 , Article 10.
Baumgardner, J. 2018. Numerical Modeling of the Large-Scale Erosion, Sediment Transport, and Deposition Processes of the Genesis Flood. Answers Research Journal 11 (2018):149–170. www.answersingenesis.org/arj/v11/numerical-modeling-sediment-transport.pdf
Baumgardner, J. 2021. How Large Tsunamis from CPT Generated the Flood Sediment Record. Midwest Creation Fellowship. Retrieved December 7, 2022 from. https://www.youtube.com/watch?v=AasMA5A21Tk
Bennett, S., Sullivan, J., 2016. The Characterisation of TLC NAND Flash Memory, Leading to a Definable Endurance/Retention Trade-Off. World Academy of Science, Engineering and Technology. International Journal of Computer and Information Engineering Vol:10, No:4, 2016.
Bercovici, D., Ricard, Y. Plate tectonics, damage and inheritance. Nature 508, 513–516 (2014). https://doi.org/10.1038/nature13072
Bokulich, A., Oreskes, N. 2017. Models in the Geosciences. Handbook of Model-Based Science, pp. 891-911
Boughton, P. 2013. Hostile wells: the borehole seismic challenge. Interview with William Wills, Geoscientist at Avalon Sciences Ltd, Somerset, UK. EngineerLive.com. Retrieved December 6 2022 from https://www.engineerlive.com/content/22907
Clarey, T. 2018. Cold Slabs Indicate Recent Global Flood. ICR News. Retrieved Dec 6 2022 from https://www.icr.org/article/cold-slabs-indicate-recent-creation
Clarey, T. 2019. Four Geological Evidences for a Young Earth. Acts & Facts, ICR.
Clarey, T. 2020. Carved In Stone, Geological Evidence of the Worldwide Flood, pp. 138-141. Institute of Creation Research.
Crawford, C., Al-Attar, D., Tromp, J., Mitrovica, J. X. 2016. Forward and inverse modelling of post-seismic deformation. Geophysical Journal International, Volume 208, Issue 2, February 2017, Pages 845–876, https://doi.org/10.1093/gji/ggw414
Czerwonka, J., Greiler M., Tilford J. (2015) Code Reviews Do Not Find Bugs: How the Current Code Review Best Practice Slows Us Down In: Proceedings of the 37th International Conference on Software–Engineering - Volume 2. ICSE ’15, 27–28.. IEEE Press, Piscataway. http://dl.acm.org/citation.cfm?id=2819009.2819015.
Cho, N., J. Baumgardner, J.A. Sherburn, and M.F. Horstemeyer. 2018. Numerical investigation of strength reducing mechanisms of mantle rock during the Genesis Flood. In Proceedings of the Eighth International Conference on Creationism, ed. J.H. Whitmore, pp. 707–730. Pittsburgh, Pennsylvania: Creation Science Fellowship.
DrillingFormulas.Com, 2014. What is the longest, deepest and largest hole ever drilled on earth?
Dumberry, M., Mandea, M. 2022. Gravity Variations and Ground Deformations Resulting from Core Dynamics. Surv Geophys 43, 5–39 (2022). https://doi.org/10.1007/s10712-021-09656-2
Duncombe, J. 2019. The Unsolved Mystery of the Earth Blobs. Eos. Retrieved December 5, 2022 from https://eos.org/features/the-unsolved-mystery-of-the-earth-blobs
Emmermann, R., Lauterjung, J. 1997. The German Continental Deep Drilling Program KTB: Overview and major results. Journal of Geophysical Research, Vol. 102, No. B8, 18179–18201.
Engheim, E. 2018. How Do We Actually Find Oil? Retrieved July 20, 2022 from https://erik-engheim.medium.com/how-do-we-actually-find-oil-4d0e58d67004
Faleide, T., A. Braathen, I. Lecomte, M. Mulrooney, I. Midtkandal, A. Bugge, S. Planke, Impacts of seismic resolution on fault interpretation: Insights from seismic modelling, Tectonophysics, Volume 816, 2021, 229008, ISSN 0040-1951, https://doi.org/10.1016/j.tecto.2021.229008.
FederalRegister.gov 2016. Aviation Training Device Credit for Pilot Certification. A Rule by the Federal Aviation Administration on 04/12/2016. Retrieved December 4, 2022 from https://www.federalregister.gov/documents/2016/04/12/2016-08388/aviation-training-device-credit-for-pilot-certification
Fundytus, N. 2022. Borehole seismic and beyond. Schlumberger Limited (SLB). Retrieved December 7, 2022 from https://www.slb.com/resource-library/interview/rpev/borehole-seismic-and-beyond
Ghosh, A., Holt, W. E., Bahadori, A. 2019. Role of Large-Scale Tectonic Forces in Intraplate Earthquakes of Central and Eastern North America. Geochemistry, Geophysics, Geosystems Research Article. AGU Journal.
Gosselet, A. Le Bégat, S. Combining borehole and surface seismic data for velocity field estimation through slope tomography, Geophysical Journal International, Volume 176, Issue 3, March 2009, Pages 897–908, https://doi.org/10.1111/j.1365-246X.2008.04022.x
Gray M., R. Bell, J. Morgan, S. Henrys, D. Barker, 2019. Imaging the Shallow Subsurface Structure of the North Hikurangi Subduction Zone, New Zealand, Using 2-D Full-Waveform Inversion. Journal of Geophysical Research: Solid Earth, Volume 124, Issue 8, Pages 9049-9074. https://doi.org/10.1029/2019JB017793
Guliuzza, R. J. 2017. Engineered Adaptability: Continuous Environmental Tracking Wrap-Up. Acts & Facts. 48 (8):17-19.
Guliuzza, R. J. 2019. Harvard Research Supports Innate Adaptive Mechanisms. News, Creation Science Update, ICR. Retrieved December 10, 2022 from https://www.icr.org/article/harvard-supports-innate-adaptive-mechanisms
Ham, K. 2021. Plate Tectonics: Creationist Idea Still Makes Accurate Predictions. Answers in Genesis, Ken Ham Blog. Retrieved December 6, 2022 from https://answersingenesis.org/geology/plate-tectonics/plate-tectonics-creationist-idea-still-makes-accurate-predictions-/
Hebert, J. 2017. The Flood, Catastrophic Plate Tectonics, and Earth History. Acts & Facts. 46(8): 11-13.
Heron, P. & Garnero, E. 2019. What lies beneath: Thoughts on the lower mantle. Geoscientist 29 (3), 10-15. https://doi.org/10.1144/geosci2019-015
Julian, B. 2006. Seismology: The hunt for plumes. Retrieved July 20, 2022 from http://www.mantleplumes.org/WebpagePDFs/Seismology.pdf
Koroni, M., Bowden, D. 2019. On the resolution of seismic tomography models and the connection to geodynamic modelling. The blog of the Geodynamics (GD) Division of the European Geosciences Union(EGU). Retrieved December 7, 2022 from https://blogs.egu.eu/divisions/gd/2019/06/05/on-the-resolution-of-seismic-tomography-models-and-the-connection-to-geodynamic-modelling-is-blue-red-the-new-cold-hot-how-many-pixels-in-an-earth/
Lightner, J. 2019. Dubious Claims About Natural Selection. Answers Research Journal 12 (2019): 41–43.
Liu, L., Cao, W., Liu, H., Ord, A., Qin, Y., Zhou, F., Bi, C. 2022. Applying benefits and avoiding pitfalls of 3D computational modeling-based machine learning prediction for exploration targeting: Lessons from two mines in the Tongling-Anqing district, eastern China, Ore Geology Reviews, Volume 142, 2022, 104712, ISSN 0169-1368, https://doi.org/10.1016/j.oregeorev.2022.104712.
Madeyski, L. 2010. The impact of Test-First programming on branch coverage and mutation score indicator of unit tests: An experiment. Information and Software Technology 52, 2 (2010)
Mark, R., 2019. The Ever-changing Landscape of Flight Simulation. Flyingmag.com. Retrieved December 3 2022 from https://www.flyingmag.com/ever-changing-landscape-flight-simulation/
Matsumura, M. 1997. Miracles In, Creationism Out. Reports of the National Center for Science Education. Volume 17, No. 3
Miftakhov, R. I reviewed 9 geophysics papers on Deep learning for Seismic INVERSE problems. Retrieved Dec 1, 2022 from https://www.youtube.com/watch?v=u94lwuzMb9M
Moresi, L. (2021). Underworld2, Computable Model, OpenGMS. Retrieved December 5, 2022 from https://geomodeling.njnu.edu.cn/computableModel/fedf931e-89ea-414f-838b-8c33a8b7ebd9
Munir, H., Moayyed, M., Petersen, K.. 2014. Considering Rigor and Relevance when Evaluating Test Driven Development: A Systematic Review. Inf. Softw. Technol. 56, 4 (April 2014), 375–394. https://doi.org/10.1016/j.infsof.2014
Notman, N. 2022. Drilling deep to discover the secrets of the mantle. Chemistry World, Royal Society of Chemistry. 14 Feb 2022.
Olson P. (editor). 2010. Grand Challenges in–Geodynamics - Outstanding geodynamics problems and emerging research opportunities for the Earth Sciences. Opportunities and Challenges in Computational Geophysics.
Pavel, M., White, M., Padfield, G., Taghizad, A. 2013. Validation of mathematical models for helicopter flight simulators past, present and future challenges. Aeronautical Journal 117(1190):343-388
Pinet, N., Gloaguen, W., Giroux, B. (2019) Introduction to the special issue on geophysics applied to mineral exploration. Canadian Journal of Earth Sciences. 56(5): v-viii. https://doi.org/10.1139/cjes-2018-0314
Popov, A., Sobolev, S. 2010. Finding a suitable finite element for 3D geodynamic modeling. Geophysical Research Abstracts Vol. 12, EGU2010-12909, 2010 EGU General Assembly 2010
Provost, A., T. Reilly, A. Harbaugh, D. Pollock. 2010. U.S. Geological Survey Groundwater Modeling Software: Making Sense of a Complex Natural Resource. USGS Fact Sheet 2009-3105.
Reid, James. 2014. Introduction to Geophysical Modelling and Inversion. Geophysical Inversion For Mineral Explorers. Retrieved 11/25/2022, from Using seismic imaging to map formations below the sea-floor | ExxonMobil.
Richter, Z. 2022. What Is the Cost of a Drill Rig? Freight Waves Ratings. Retrieved December 6 2022 from https://ratings.freightwaves.com/
Saastamoinena, K., Maunulab, K. 2021. Usefulness of flight simulator as a part of military pilots training –case study: Grob G 115E. Procedia Computer Science 192 (2021) 1670–1676
Sanford, J., Baumgardner, J., Gibson, P., Brewer, W., & ReMine, W. (2008). Using Numerical simulation to test the validity of neo-Darwinian theory. In A.A. Snelling (Ed.), Proceedings of the sixth international conference on creationism (pp. 165–175). Pittsburgh, Pennsylvania: Creation Science Fellowship and Dallas, Texas: Institute for Creation Research.
Scales, J., M. Smith, S. Treitel. 2001. Introductory Geophysical Inverse Theory. Samizdat Press, Department of Geophysics Colorado School of Mines.
Schlumberger. 2014. Elastic Full-Waveform Inversion (PDF, slb.com). Accessed 11/30/2022.
Schmandt, B., Lin, F. 2014. P and S wave tomography of the mantle beneath the United States. Geophysical Research Letters. 41: 6342-6349.
Stierwalt, S. 2020. How Deep Is the Deepest Hole in the World? Scientific American. Retrieved December 20, 2022 from https://www.scientificamerican.com/article/how-deep-is-the-deepest-hole-in-the-world/
van Zelst, I., Crameri, F., Pusok, A. E., Glerum, A., Dannberg, J., and Thieulot, C. 2022. 101 geodynamic modelling: how to design, interpret, and communicate numerical studies of the solid Earth. Solid Earth, 13, 583–637, https://doi.org/10.5194/se-13-583-2022.
Williams F., 2005. Tom Schneider’s “the And-multiplication Error” Article Refuted. Retrieved December 7, 2022 from https://tinyurl.com/4duxa2tw.
Zaccagnino, D., Doglioni, C. 2022. Earth’s gradients as the engine of plate tectonics and earthquakes. Riv. Nuovo Cim. 45, 801–881 (2022). https://doi.org/10.1007/s40766-022-00038-x
Zhang, X., X. Song, J. Li. 2022. A comparative study of seismic tomography models of the Chinese continental lithosphere. Earthquake Science, Volume 35, Issue 3, 2022, Pages 161-185, ISSN 1674-4519, https://doi.org/10.1016/j.eqs.2022.05.005.
Zhao D., Xu Y., Wiens DA., Dorman L., Hildebrand J., Webb, S. 1997. Depth extent of the Lau back-arc spreading center and its relation to subduction processes. Science 278:254–257
Zhu, H., Stern, R.J. & Yang, J. 2020. Seismic evidence for subduction-induced mantle flows underneath Middle America. Nature Communications 11, 2075 (2020). https://doi.org/10.1038/s41467-020-15492-6
Figure 1. P-Wave tomography of Tonga trench (Zhao 1997).
Figure 2. Tomography images of “inferred” slab remnants (Schmandt 2014).
Figure 3. 3D imaging of plates centered around Central America (Zhu 2020).
Figure 4. Seismic tomography images attributed to Alessandro Forte, 2003.
Figure 5. Anonymous Seismologist. 2019. Visualization of low-velocity anomalies beneath the Pacific Ocean. Retrieved Dec 1, 2022, from https://www.youtube.com/watch?v=NQe8hwVtirM
Figure 6. The GeoDynamic modeling lifecycle (used with permission by Iris van Zelst 2021).